GPTMB 2025 - The Second International Conference on Generative Pre-trained Transformer Models and Beyond
July 06, 2025 - July 10, 2025
GPTMB 2025
ISSN:
ISBN: 978-1-68558-287-6
GPTMB 2025 is colocated with the following events as part of DigiTech 2025 Congress:
- DIGITAL 2025, Advances on Societal Digital Transformation
- IoTAI 2025, The Second International Conference on IoT-AI
- GPTMB 2025, The Second International Conference on Generative Pre-trained Transformer Models and Beyond
GPTMB 2025 Steering Committee
|
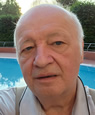 |
Petre Dini
IARIA
USA/EU
|
|
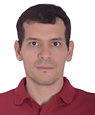 |
Isaac Caicedo-Castro
University of Córdoba
Colombia
|
|
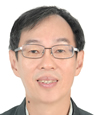 |
Tzung-Pei Hong
National University of Kaohsiung
Taiwan
|
|
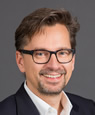 |
Stephan Böhm
RheinMain University of Applied Sciences - Wiesbaden
Germany
|
|
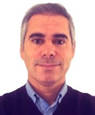 |
Alper Yaman
Fraunhofer IPA
Germany
|
|
|
|
GPTMB 2025 conference tracks:
Generative-AI basics
Generative Pre-trained Transformer Models
Combination of GPT models and Reinforcement learning models
Creativity and originality in GPT-based tools
Deep learning
Large Language Models (LLM)
Autoregressive language models
Accuracy and relevancy
Critical issues on Input Data
Datasets: accuracy, granularity, precision, false/true negative/positive
Visible vs invisible (private, personalized) data
Data extrapolation
Output biases and biased Datasets
Sensitivity and specificity of Datasets
Fake and incorrect information
Volatile data
Time sensitive data
Critical Issues on Processing
Process truthfulness
Explainability
Detect biases and incorrectness
Incorporate the interactive feedback
Incorporate corrections
Output quality
Output biases and biased Datasets
Sensitivity and specificity of Datasets
Context-aware output
Fine/Coarse text summarization
Quality of Data pre-evaluation (obsolete, incomplete, fake, noisy, etc.)
Validation of output
Detect biased and incorrect summarization before spreading it
Education and academic liability issues
User awareness on output trust-ability
Copyright infringements rules
Plagiarism and self-plagiarism tools
Ownership infringement
Mechanisms for reference verification
Dealing with hidden self-references
Regulations and limitations
Regulations (licensing, testing, compliance-threshold, decentralized/centralize innovations)
Mitigate societal risks of GPT models
Capturing emotion and sentience
Lack of personalized (individual) memory and memories (past facts)
Lack of instant personalized thinking (personalized summarization)
Risk of GPTM-based decisions
AI awareness
AI-induced deskilling
Case studies with analysis and testing AI applications
Lesson learned with existing tools (ChatGPT, Bard AI, ChatSonic, etc.)
Predictive analytics in healthcare
Medical Diagnostics
Medical Imaging
Pharmacology
AI-based therapy
AI-based finance
AI-based planning
AI-based decision
AI-based systems control
AI-based education
AI-based cyber security
Deadlines:
Submission | Mar 18, 2025 |
Notification | May 04, 2025 |
Registration | May 18, 2025 |
Camera ready | Jun 01, 2025 |
Deadlines differ for special tracks. Please consult the conference home page for special tracks Call for Papers (if any).